Mercedes-Benz officially announced in mid-June that it will integrate its car voice assistant with ChatGPT. This marks the first time ChatGPT has been used in a car environment. With ChatGPT’s integration, significant changes are expected for the intelligent automotive operating system. In this article, we will analyze the changes that artificial intelligence (AI) will bring to the automotive industry.
On June 16th, Mercedes-Benz officially announced the integration of its car voice assistant with ChatGPT. Over 900,000 American customers can now participate in the MBUX intelligent human-machine interaction system test plan through the Mercedes me app or the “Hey, Mercedes” voice command.
The automobile industry provides the largest interactive application scenario for big models, and this marks the first time ChatGPT has been used in a car environment. The results of this test will be used to further improve the voice assistant and provide reference for large language models in more markets. With ChatGPT’s integration, the intelligent automotive operating system will also be reshaped.
Apart from Mercedes-Benz, many other companies are also exploring and focusing on the application of big models in cars, including Tesla, Nvidia, Baidu, and Alibaba. The combination of AI and automobiles is an urgent development trend both domestically and internationally, and no company wants to fall behind in this new revolution led by AI.
In the midst of everyone discussing AI today, we also want to briefly discuss how artificial intelligence will bring changes to the automotive industry.
An intelligent cockpit that understands you better.
The emergence of big models has brought new opportunities and challenges to the development of AI, as well as new possibilities for the automotive industry, particularly in the areas of intelligent cockpits and autonomous driving.
An intelligent cockpit is a car interior space that integrates various intelligent functions and services. It can provide a safe, comfortable, convenient, and enjoyable travel experience for passengers through multimodal human-vehicle interaction.
The application of big models in intelligent cockpits is mainly reflected in voice interaction.
Voice interaction is one of the most important functions in an intelligent cockpit. It allows passengers to communicate and control the car through natural language, improving the convenience and safety of travel.
According to data from AutoVision Research Institute, a research institution for automotive intelligence, by 2023, the proportion of car models equipped with voice interaction function at the Shanghai Auto Show will reach 95%, with Mercedes-Benz ranking first in terms of voice interaction.
One of the reasons why Mercedes-Benz leads in voice interaction is due to its use of ChatGPT technology. Mercedes-Benz has integrated ChatGPT into its MBUX intelligent human-machine interaction system, providing car owners with a new voice assistant experience. The system supports more dynamic conversations, accurately understands voice commands, and engages in interactive dialogues with car owners.
Another important aspect is image analysis. Image big models can provide facial recognition, emotion analysis, AR camera, and other services for intelligent cockpits, allowing passengers to interact and entertain with the car through images. For example, SenseMirage, an image generation model released by SenseTime, can generate images of various styles and themes based on the input or selection of the car owner.
In addition to voice interaction and image analysis, 3D content big models can also empower intelligent cockpits by providing 3D navigation services and more.
In summary, the application of big models in intelligent cockpits can provide cars with stronger perception, understanding, generation, and interaction capabilities, thereby providing passengers with a more intelligent, personalized, and scenario-based travel experience.
This is an ongoing interactive revolution, and this revolution cannot be separated from another important development direction of the future transportation industry – autonomous driving.
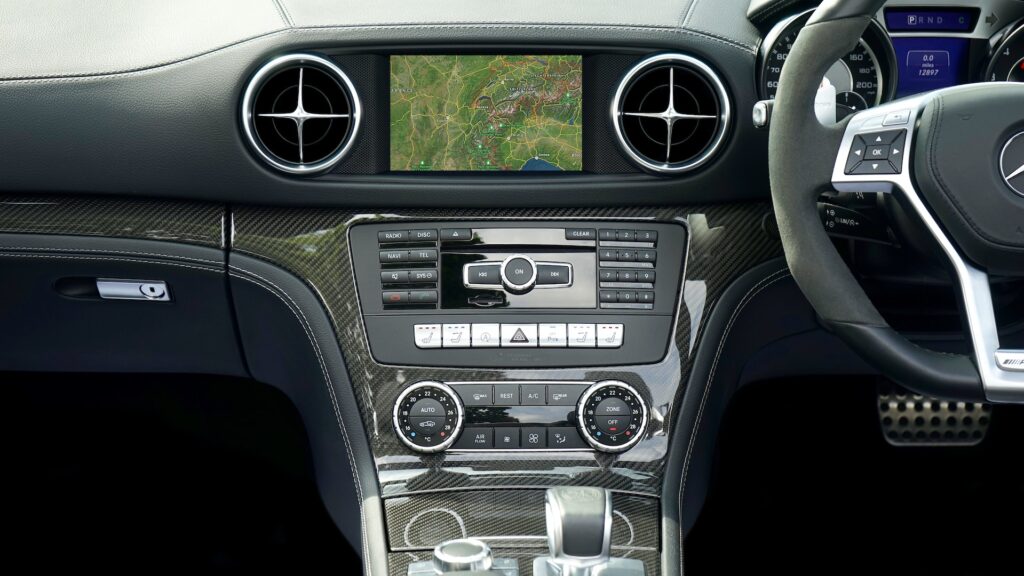
Accelerating the development of autonomous driving.
Autonomous driving technology is an important development direction for the future transportation industry, and AI is one of the key technologies to achieve it. Autonomous driving refers to the technology that allows vehicles to replace human drivers to different extents through computer systems’ perception, decision-making, and execution.
According to the standard of the Society of Automotive Engineers (SAE), autonomous driving can be divided into six levels from L0 to L5, representing different levels of automation and human-machine interaction methods. L0-L2 belong to driver assistance, while L4-L5 are considered autonomous driving.
Currently, the highest level we have achieved is L3, which means that with the help of artificial intelligence, vehicles can handle all driving tasks, but the driver still needs to be present in case of emergencies or similar system failures.
Autonomous driving L0-L5:
- L0: Manual driving;
- L1: Driver assistance, where the car can provide some assistance functions, but the driver still needs to monitor the surroundings and be ready to take control of the vehicle at any time;
- L2: Partial autonomous driving, where the car can provide multiple assistance functions, such as an advanced driver assistance system (ADAS) that combines AIGC and LKA;
- L3: Conditional autonomous driving, where the vehicle can complete all driving operations and monitor the surrounding environment in specific scenarios and conditions, but appropriate responses must be provided by humans when the system requires it.
- L4: Highly automated driving, where the vehicle can complete all driving operations and monitor the surrounding environment under specific scenarios and conditions, such as unmanned logistics, unmanned micro-buses, and autonomous parking, without requiring any response from humans. At this time, there is no need to have a safety driver in the car, but road and environmental conditions are still limited.
- L5: Fully autonomous driving, where there is no need to have a safety driver in the car and road and environmental conditions are not limited.
The principle of technology.
Autonomous driving utilizes advanced information technologies such as computer-based machine learning, high-precision mapping, positioning, network communication, and LiDAR to enable vehicles to effectively control and avoid road conditions, potential obstacles, and hazards during automatic driving. It adopts techniques such as environmental perception, automatic decision-making, and control to take effective measures against various complex environments and unexpected situations. Its basic principles include perception, decision-making, and control.
Perception is the foundation of autonomous driving and the prerequisite for decision-making and control. It precisely identifies the surrounding environment of the vehicle through millimeter-wave radar, LiDAR, and cameras, autonomously avoids obstacles, and performs automatic turning.
Decision-making refers to the vehicle’s planning and judgment based on perceived information through intelligent algorithms and models to determine the appropriate working mode and control strategy in place of human driver decision-making. Decision-making mainly relies on chips and software, is the core of autonomous driving, and includes path planning, behavior planning, trajectory planning, and interaction planning.
Control is the realization of autonomous driving and the result of perception and decision-making. It refers to the vehicle’s operation based on the output of the decision through wire control or mechanical systems, such as turning, accelerating, decelerating, and braking.
Control mainly includes two aspects: longitudinal control and lateral control. The former controls the speed and acceleration of the vehicle in the direction of travel, mainly involving the throttle and brake system; the latter controls the position and angle of the vehicle perpendicular to the direction of travel, mainly involving the steering system.
The application domain.
The significant advancement in artificial intelligence has greatly improved the capability of autonomous driving systems. By combining machine learning algorithms, computer vision, and sensor fusion technology, these systems can understand the surrounding environment and respond to it, becoming more reliable, efficient, and safe.
The main focus in utilizing artificial intelligence to achieve a thorough change in driving is on the application domain of autonomous driving, which includes environment perception, decision-making, and learning adaptation.
Autonomous vehicles combine cameras, LiDAR, radar, and other sensors to collect data about the surrounding environment. Artificial intelligence algorithms then process this data to create a detailed map of the environment and identify objects, such as pedestrians, other vehicles, traffic lights, and road signs, to determine how the vehicle should respond. High-speed storage such as GDDR6 can support fast data storage and access, enabling intensive computing.
Autonomous cars use artificial intelligence to make real-time decisions based on the data collected from sensors. For example, if an autonomous car detects a pedestrian crossing the road, it will use artificial intelligence to determine the best course of action, such as slowing down or stopping.
At the same time, predictive modeling and supervised learning algorithms can predict the behavior of other road users, such as pedestrians crossing the road at specific locations and other vehicles changing lanes. This helps the car predict potential traffic issues and take appropriate action to avoid them.
Unsupervised learning algorithms can be used to identify anomalies or unexpected events in the data collected by autonomous vehicle sensors, such as pedestrians crossing the road at unexpected locations or vehicles suddenly changing lanes.
Autonomous driving systems can also continuously improve their performance through machine learning and deep learning techniques. By constantly collecting and analyzing driving data, the system can learn and adapt to different driving scenarios, improving its decision-making capabilities and response times.
Furthermore, reinforcement learning techniques also play an important role in the field of autonomous driving. Through reinforcement learning, vehicles can continuously trial-and-error and learn in real-world driving environments, optimizing their driving strategies and decision-making abilities.
What direction is the future headed?
In contemporary society, cars are an essential means of transportation for human beings and an economic engine for social development. With the continuous advancement of artificial intelligence technology, autonomous driving technology will gradually become commercialized and further popularized.
There is also a viewpoint that cars are expected to become part of the intelligent mobile terminal. The development of artificial intelligence and the internet provides cars with the ability to interact with other intelligent mobile terminals. Our intelligent life will also become more expandable and unique because of the mobile private space property of cars.
As the world’s highest-valued car company, Tesla is vigorously promoting autonomous driving, and it is bound to take autonomy to the end. Baidu CEO Robin Li also said that normal car accidents that happen all the time are not news, but autonomous driving accidents have become news, and the fundamental reason is that autonomous driving has not yet become popular.
Of course, the smart driving industry chain still needs to address the existing problems of policy, cost, technology, insurance, and other factors. However, the future of autonomous driving technology is bright, and we look forward to a more intelligent and safe travel life.